Lecture 17: Quasi-Newton methods#
The problem#
Given a continuous function \(f(x)\), the problem is to find a point \(x^*\) such that \(f(x^*) = 0\). That is, \(x^*\) is a solution of the equation \(f(x) = 0\) and is called a root of \(f(x)\).
Recall Newton’s method:#
def newton(f, df, x0, tol=1.0e-10):
x = x0
while abs(f(x)) > tol:
x = x - f(x) / df(x)
return x
Newton’s method requires function and it’s derivative.
This may not be possible since:
\(f(x)\) may be a “black box”
the formula for \(f(x)\) may be known but difficult to differentiate
the derivative maybe very expensive to evaluate
Approximating f’(x)#
Let’s approximate \(f'(x)\) like we approximated \(y'(t)\) when solving a differential equation!
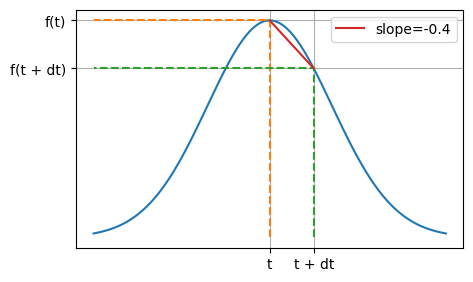
Modified Newton’s method#
Recall that \(f'(x) = \lim_{\mathrm{d}x \to 0} \frac{f(x + \mathrm{d}x) - f(x)}{\mathrm{d}x}\).
Hence we can choose a small value for \(\mathrm{d}x\) (how small?) and approximate:
\[ f'(x) \approx \frac{f(x + \mathrm{d}x) - f(x)}{\mathrm{d}x}. \]This modified-Newton method then becomes
\[ x^{(i+1)} = x^{(i)} - \frac{\mathrm{d}x \times f(x^{(i)})}{f(x^{(i)} + \mathrm{d}x) - f(x^{(i)})}. \]
def modified_newton(f, x0, dx, tol=1.0e-10):
x = x0
while abs(f(x)) > tol:
df = (f(x + dx) - f(x)) / dx # two extra evaluations of f
# per iteration
x = x - f(x) / df
return x
def modified_newton2(f, x0, dx, tol=1.0e-10):
x = x0
fx = f(x)
while abs(fx) > tol:
df = (f(x + dx) - fx) / dx # one extra evaluations of f
# per iteration
x = x - fx / df
fx = f(x) # one original evaluation of f per iteration
return x
Graphical representation of modified Newton#
<>:17: SyntaxWarning: invalid escape sequence '\m'
<>:18: SyntaxWarning: invalid escape sequence '\m'
<>:17: SyntaxWarning: invalid escape sequence '\m'
<>:18: SyntaxWarning: invalid escape sequence '\m'
/tmp/ipykernel_2269/244923238.py:17: SyntaxWarning: invalid escape sequence '\m'
plt.xticks([x0, x0 + dx, x1], ["$x^{(0)}$", "$x^{(0)} + \mathrm{d}x$", "$x^{(1)}$"])
/tmp/ipykernel_2269/244923238.py:18: SyntaxWarning: invalid escape sequence '\m'
plt.yticks([f(x0), f(x0 + dx)], ["$f(x^{(0)})$", "$f(x^{(0)} + \mathrm{d}x)$"])
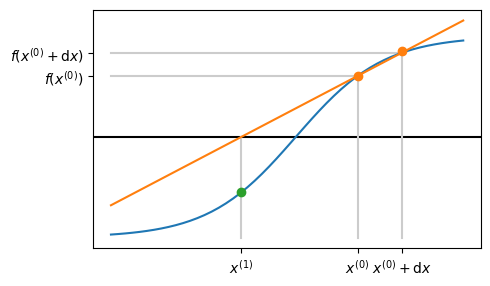
How to choose \(\mathrm{d}x\)?#
Smaller - more accurate approximation
Large - too small and we will have rounding problems (subtracting two similar numbers)
Example#
When \(f(x) = x^3\) then \(f'(x) = 3 x^2\).
Hence, at \(x_0 = 1\), \(f(x_0) = 1\) and \(f'(x_0) = 3\).
Consider what happens when we approximate this with python, using finite values for \(\mathrm{d}x\).
dx | approx | abs error | rel error |
---|---|---|---|
1.000000e-04 | 3.000300 | 3.000100e-04 | 1.000033e-04 |
1.000000e-06 | 3.000003 | 2.999798e-06 | 9.999326e-07 |
1.000000e-08 | 3.000000 | 3.972048e-09 | 1.324016e-09 |
1.000000e-10 | 3.000000 | 2.482211e-07 | 8.274037e-08 |
1.000000e-12 | 3.000267 | 2.667017e-04 | 8.890058e-05 |
1.000000e-14 | 2.997602 | 2.397834e-03 | 7.992778e-04 |
1.000000e-16 | 0.000000 | 3.000000e+00 | 1.000000e+00 |
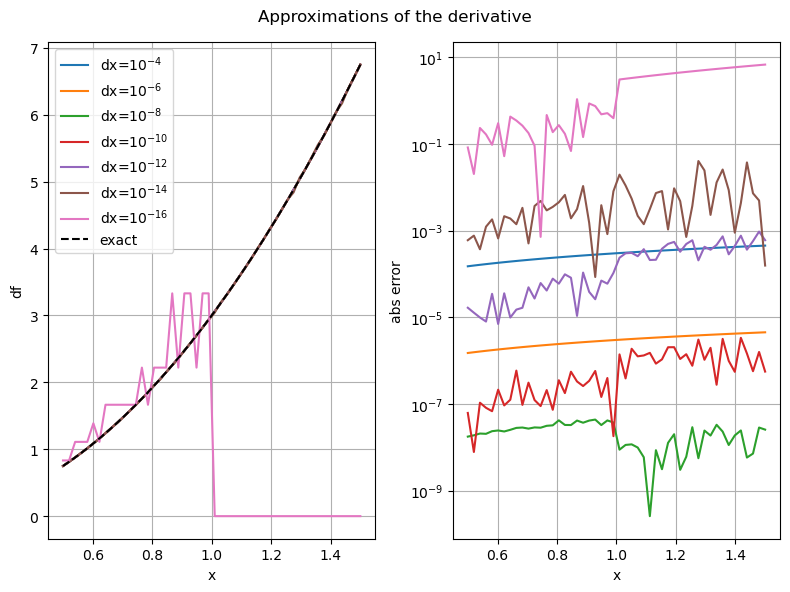
A special choice!#
Recall the definition of machine precision/unit roundoff from Lecture 3.
The modified Newton method uses \(\mathrm{d}x = \sqrt{eps}\).
eps = np.finfo(np.double).eps
dx = np.sqrt(eps)
print(f"{eps=} {dx=}")
eps=2.220446049250313e-16 dx=1.4901161193847656e-08
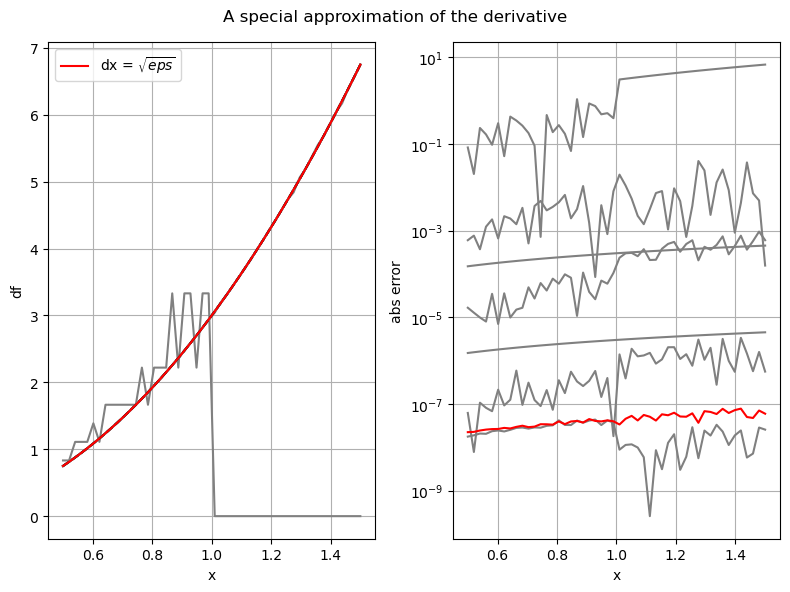
Example#
Recall the NACA0012 aerofoil example:
def f_naca0012(x):
t = 0.1
yp = (
-0.1015 * x**4 + 0.2843 * x**3 - 0.3516 * x**2 - 0.126 * x + 0.2969 * np.sqrt(x)
)
f = yp - 0.5 * t
return f
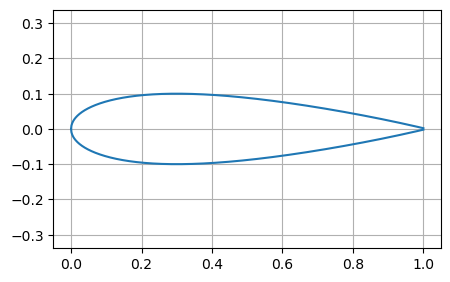
Starting from \(x^{(0)} = 1\) with \(TOL = 10^{-4}\), we get the root as \(x^* \approx 0.765789\) after 2 iterations for the NACA0012 aerofoil example (Same as Newton!):
modified_newton_with_table(f_naca0012, x0=1.0, tol=1.0e-4)
iter | x | f(x) |
---|---|---|
0 | 1.000000 | -0.047900 |
1 | 0.795168 | -0.005392 |
2 | 0.765789 | -0.000096 |
Starting from \(x^{(0)} = 0.1\) with \(TOL = 10^{-4}\), we get the root as \(x^* \approx 0.033863\) after 5 iterations for the second solution to the NACA0012 aerofoil example (Same as Newton!).
modified_newton_with_table(f_naca0012, x0=0.1, tol=1.0e-4)
iter | x | f(x) |
---|---|---|
0 | 0.100000 | 0.028046 |
1 | 0.000278 | -0.045086 |
2 | 0.005413 | -0.028849 |
3 | 0.020693 | -0.010046 |
4 | 0.031958 | -0.001300 |
5 | 0.033863 | -0.000024 |
The secant method#
Can we use a similar derivative approximation but avoid the extra function evaluation?
Recall modified-Newton method:
Now with \(\mathrm{d}x = x^{(i)} - x^{(i-1)}\) becomes
def secant(f, x0, x1, tol=1.0e-10):
# two initial function evaluations
f0 = f(x0)
f1 = f(x1)
while abs(f1) > tol:
# compute derivative approximation
df = (f1 - f0) / (x1 - x0)
# update x
x2 = x1 - f1 / df
# update other variables
x0, f0 = x1, f1
x1, f1 = x2, f(x2) # one evaluation of f per iteration
return x1
A geometric interpretation#
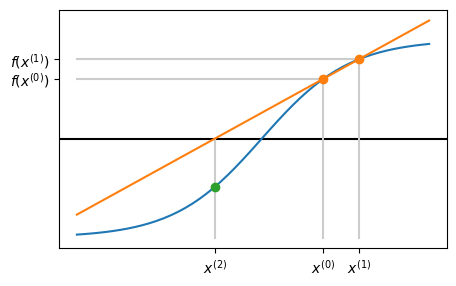
Numerical examples#
The naca0012 example starting from 1 and 0.9 to a tolerance of \(10^{-4}\) gives the solution as \(x^* \approx 0.765264\) after 3 iterations (one more than Newton!)
secant_with_table(f_naca0012, x0=1.0, x1=0.9, tol=1.0e-4)
iter | x0 | f(x0) | x1 | f(x1) |
---|---|---|---|---|
0 | 1.000000 | -0.047900 | 0.900000 | -0.025871 |
1 | 0.900000 | -0.025871 | 0.782556 | -0.003095 |
2 | 0.782556 | -0.003095 | 0.766598 | -0.000239 |
3 | 0.766598 | -0.000239 | 0.765264 | -0.000003 |
Starting from \(x^{(0)} = 0.0\) and \(x^{(1)} = 0.1\) with \(TOL = 10^{-4}\), we get the root as \(x^* \approx 0.033870\) after 5 iterations for the second solution to the NACA0012 aerofoil example (Same as Newton!).
secant_with_table(f_naca0012, x0=0.0, x1=0.1, tol=1.0e-4)
iter | x0 | f(x0) | x1 | f(x1) |
---|---|---|---|---|
0 | 0.000000 | -0.050000 | 0.100000 | 0.028046 |
1 | 0.100000 | 0.028046 | 0.064065 | 0.015706 |
2 | 0.064065 | 0.015706 | 0.018327 | -0.012232 |
3 | 0.018327 | -0.012232 | 0.038352 | 0.002810 |
4 | 0.038352 | 0.002810 | 0.034611 | 0.000465 |
5 | 0.034611 | 0.000465 | 0.033870 | -0.000019 |
Turning points?#
def f_turning(x):
return (x - 1) ** 2
secant_with_table(f_turning, x0=4.0, x1=3.0, tol=1.0e-4)
iter | x0 | f(x0) | x1 | f(x1) |
---|---|---|---|---|
0 | 4.000000 | 9.000000 | 3.000000 | 4.000000 |
1 | 3.000000 | 4.000000 | 2.200000 | 1.440000 |
2 | 2.200000 | 1.440000 | 1.750000 | 0.562500 |
3 | 1.750000 | 0.562500 | 1.461538 | 0.213018 |
4 | 1.461538 | 0.213018 | 1.285714 | 0.081633 |
5 | 1.285714 | 0.081633 | 1.176471 | 0.031142 |
6 | 1.176471 | 0.031142 | 1.109091 | 0.011901 |
7 | 1.109091 | 0.011901 | 1.067416 | 0.004545 |
8 | 1.067416 | 0.004545 | 1.041667 | 0.001736 |
9 | 1.041667 | 0.001736 | 1.025751 | 0.000663 |
10 | 1.025751 | 0.000663 | 1.015915 | 0.000253 |
11 | 1.015915 | 0.000253 | 1.009836 | 0.000097 |
Other problems#
Even more care is required to avoid divide by zero errors
# equal function values at x0 and x1
secant(f_turning, x0=4.0, x1=-2.0, tol=1.0e-4)
---------------------------------------------------------------------------
ZeroDivisionError Traceback (most recent call last)
Cell In[23], line 2
1 # equal function values at x0 and x1
----> 2 secant(f_turning, x0=4.0, x1=-2.0, tol=1.0e-4)
Cell In[17], line 10, in secant(f, x0, x1, tol)
8 df = (f1 - f0) / (x1 - x0)
9 # update x
---> 10 x2 = x1 - f1 / df
12 # update other variables
13 x0, f0 = x1, f1
ZeroDivisionError: float division by zero
# too small tolerance means x0 = x1!
secant(f_turning, x0=4.0, x1=3.0, tol=1.0e-50)
---------------------------------------------------------------------------
ZeroDivisionError Traceback (most recent call last)
Cell In[24], line 2
1 # too small tolerance means x0 = x1!
----> 2 secant(f_turning, x0=4.0, x1=3.0, tol=1.0e-50)
Cell In[17], line 8, in secant(f, x0, x1, tol)
4 f1 = f(x1)
6 while abs(f1) > tol:
7 # compute derivative approximation
----> 8 df = (f1 - f0) / (x1 - x0)
9 # update x
10 x2 = x1 - f1 / df
ZeroDivisionError: float division by zero
Exercise (hard!)#
Find a periodic iteration for the secant method.
Summary#
Bisection |
Newton’s method |
Modified Newton |
Secant |
|
---|---|---|---|---|
Simple algorithm |
yes |
yes |
yes |
yes |
Starting values |
bracket |
one |
one |
two |
Iterations |
lots |
normally fewer |
similar to Newton |
similar to Newton |
Function evals |
one per iteration |
|
two per iteration |
one per iteration |
Convergence |
with good bracket |
not always |
not always |
not always |
Turing point roots |
no |
slower |
slower |
slower |
Use of derivative |
no |
yes |
no |
no |
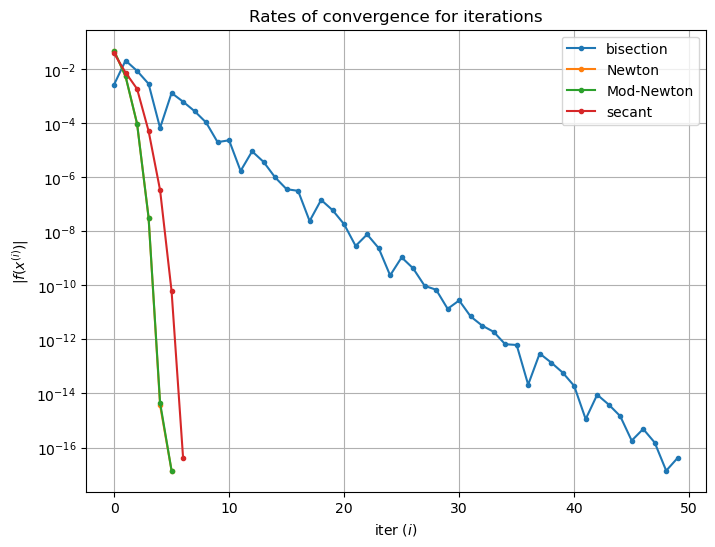
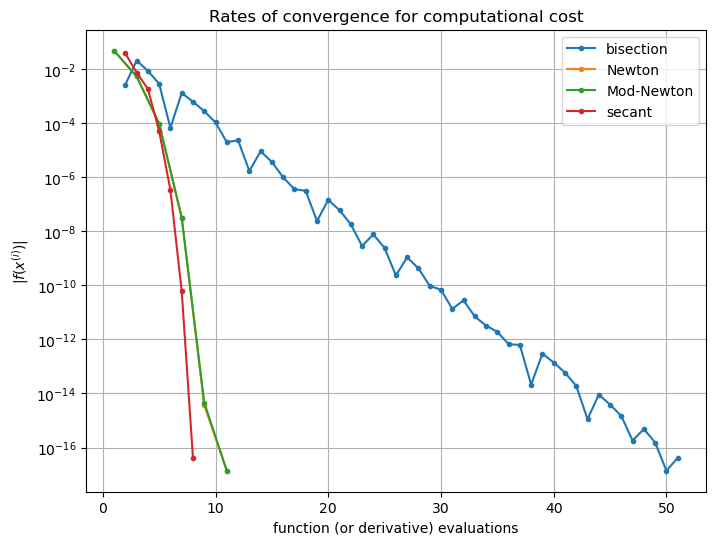